Preparing for turmoil with GaR: a new model to develop stress test scenarios for banking sector
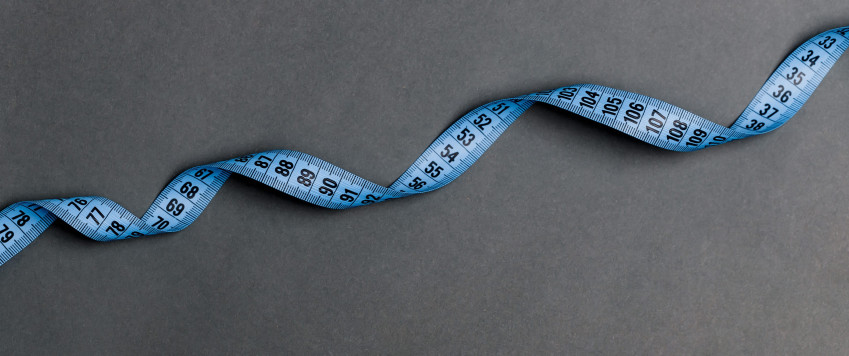
In short
-
Macroeconomic stress tests are used to assess the resilience of the banking sector to severe but plausible shock events.
-
Stress tests show whether credit institutions have sufficient capital to absorb potential losses that could arise under extreme financial stress.
-
Since 2023, Latvijas Banka has been developing a stress scenario by using the GaR (growth-at-risk) model to evaluate the potential GDP growth rate.
Latvijas Banka has been publishing stress tests of the banks' loan portfolios in the Financial Stability Report since 2004. Stress tests are a set of techniques used to assess the resilience of banks to significant macroeconomic changes or extreme but plausible events. Stress test results indicate the most significant risks and allow an assessment of the potential losses associated with them. The stress tests included a sensitivity analysis to determine the impact of changes in a single risk factor and a scenario analysis to evaluate the consequences of simultaneous changes in several risk factors.
Latvijas Banka's stress testing methodology has evolved over time. A credit risk model that links macroeconomic developments to the quality of the banking sector's loan portfolio was developed for macroeconomic stress testing in 2009 and modified in 2014. Thus, the macroeconomic credit risk model allows evaluating, how the share of loans past due over 90 days could change in the residential loan portfolio of banks under specific macroeconomic scenarios.
Latvijas Banka's macroeconomic model was used to develop stress scenarios until 2023. In the scenario development process, different shocks, such as a fall in foreign demand, a decrease in investment or a contraction of private consumption, were applied to Latvijas Banka's macroeconomic model, resulting in GDP growth rates required for adverse (stress) scenarios.
The calibration of shocks was initially based on the macroeconomic developments observed during the global financial crisis. Over time, however, the structure of Latvian economy has changed, altering the response of the economy to various shocks. It made the calibration of new shocks more challenging, raising questions:
- What would be the right combination of shocks and their magnitude in each stress test?
- What would be the probability of the combined shocks (and hence the scenario) materialising, as determining this probability in the case of multiple shocks is a very complex task?
GDP growth plays a significant role in the macroeconomic credit risk model as a proxy for borrowers' income and the phase of the economic cycle. Therefore, it was decided in 2023 to calibrate the GDP development in a stress scenario using the GaR (growth-at-risk) model instead of macroeconomic shocks.
Using the GaR model for scenario development has several advantages over the previous approach. First, GaR allows modelling theoretically possible GDP growth rate values several periods ahead depending on the state of the business cycle. This GaR feature allows scenarios to be constructed with a pre-specified probability (e.g., 1%, 5% or 10%) that determines the severity of the scenario [1]. Second, the use of GaR does not require identifying specific shocks that trigger the GDP evolution in question.
GaR model – what sort of animal is it?
Before we proceed with the GaR model and its results, let us consider a simple illustration (Figure 1). It shows the most likely GDP growth rates (close to the mean GDP growth) and the less likely ones (on the left or right tail of the distribution curve). If the largest part of the growth rates can be considered as normal growth, then the values on the left tail of the distribution curve are associated with financial stress. The lowest 5% or 10% GDP growth rates are most often considered risky (probability q in Figure 1). GaR is a future GDP growth rate that indicates financial stress at a given probability, represented by the chosen percentile [2] (5% or 10%). In other words, GaR is the threshold value for a future GDP growth rate that characterises weak GDP growth – theoretically possible but unlikely. To put it differently, at 5% GaR, GDP growth will be above 5% GaR throughout the rest of the 95% distribution. At the same time, a more pessimistic GDP growth scenario than 5% GaR is possible with a probability lower than 5%.
What would this figure look like in the case of Latvia?
Figure 2 shows that the 5th percentile corresponds to the GDP growth rate of -10%, but the 10th percentile – to a GDP growth rate close to -4%. In the second quarter of 2020 when we faced various limitations due to Covid-19, the GDP growth rate almost reached the 5th percentile of the GDP growth distribution. Meanwhile, we experienced even lower GDP growth for several consecutive quarters during the Global Financial Crisis.
So, does this mean that we can engrave these data in our memory and use them in all the future risk assessments by simply choosing the 5th or the 10th percentile?
Not exactly. It would be hard to imagine a scenario where all future crises would be equally severe irrespective of the growth observed in the past. Accordingly, the values of the GDP growth rate at risk have to be different in different phases of the business cycle. We should also consider other factors that can influence the expected GDP values. Several research papers [3] note that the financial stress indicators significantly influence the probability distribution of the GDP growth rate.
How exactly can we determine the lowest realistic GDP growth rate values that we can use in risk assessment?
Now is the right time to turn back to the GaR model. It allows evaluating the probability distribution of GDP growth rates (usually the values of particular percentiles of this distribution) one, two, three etc. periods ahead, taking into account the past GDP dynamics and factors used in the model. Currently, Latvijas Banka needs GDP forecasts for the next calendar year [4] to conduct its stress tests.
Evaluation of different GaR models led to the conclusion that the models with additional factors give more accurate projections of the GDP growth rate at risk than models with the GDP growth rate as the only factor [5]. Therefore, the GaR model includes the deflated composite cyclical risk indicator (deflated CCRI) as an additional factor for the purposes of stress testing. The CCRI has been developed based on the ECB methodology; it combines indicators that provide early warning signals for crisis and characterises risks specific to Latvia. The indicators included in the CCRI cover housing prices, lending dynamics, external imbalances, resilience of credit institutions and private sector debt burden.
Calculation results (Figure 3) indicate that the GaR value will continue to decrease and will fall below the historical 10th percentile; moreover, it might even approach the 5th percentile in the second half of 2024. We will, however, see an upward trend at the end of 2024. If the GaR projections were to materialise, annual GDP would fall by 6.8% in line with the 10th percentile GaR and by 8.0% in line with the 5th percentile GaR in 2024.
Should we give up and prepare for the worst?
No way! The objective of the GaR model is not to calculate the most accurate forecasts. On the contrary, this is about the severe but plausible scenarios that are so important in stress testing. After all, one of the tasks of Latvijas Banka is to ensure financial stability of the Latvian financial system. Therefore, it is essential to understand in good time whether, for example, a credit institution might have problems meeting its minimum capital requirements under exceptional circumstances as risks materialise.
Methodology and technical details
Three indicators were selected as the most appropriate to characterise developments and risks in the financial sector – the deflated CCRI, the credit-to-GDP gap and the change in the ratio of loans to deposits. Values of all these indicators were normalised before the model estimation – recalculated so that their means are 0 and the standard deviation is 1. Accordingly, three GaR model groups were estimated, each of them containing the GDP growth rate and one of the indicators characterising the financial cycle.
Since the beginning of the Covid-19 crisis was not related to unfavourable developments in economics of the financial sector, the most appropriate model estimation period was chosen as the first step. The results of the GaR models were compared using all available data, all data combined with the variables [6] that exclude the second and third quarters of 2020 from the calculations and using the data until the end of 2019 (pre-Covid-19 period). Based on the pseudo coefficient of determination (R2) criterion, the models with the variables that exclude the Covid-19 crisis were used as the most appropriate ones.
Currently, Latvijas Banka requires GDP forecasts for up to six quarters into the future for its stress tests. Therefore, each set of models was estimated sequentially for six future periods. A comparison of the 95% confidence intervals of the coefficients for the 5th percentile GaR models is illustrated in Figure 4, but that for the 10th percentile GaR models – in Figure 5. Both figures show that indicators characterising the financial cycle negatively influence the GDP growth rate at risk. Also, we can see that, in most cases, the coefficients of indicators characterising the financial cycle become statistically significant from the third future period onwards. These observations can be justified by the nature of the financial cycle indicators. Firstly, positive values of these indicators signal the build-up of risks; it is, therefore, obvious that higher risk implies lower GDP growth values. Secondly, financial cycle indicators can signal an approaching crisis several years in advance (Chen & Svirydzenka, 2021); therefore, it is logical that these indicators become more informative in longer term.
It should be noted that the analysis of the model accuracy was carried out using both in-sample and pseudo out-of-sample procedures (65% of the sample is used for the initial calculations and then the sample is extended by 1 quarter at a time, assessing the accuracy of the predictions against the actual out-of-sample data at each step). Almost in all cases, the results of the analysis show that the GaR models with financial cycle indicators estimate the GDP growth rate at risk more accurately than the GaR model that is based on GDP alone, except for the model with the credit-to-GDP gap (for some horizons), which was the first to be excluded from the selection of models. The results of models, which included the deflated CCRI and the loan-to-deposit ratio, were similarly accurate. As the deflated CCRI covers a broader range of indicators and thus more versatile aspects of potential risks, it was chosen to be included in the GaR model as the most appropriate indicator characterising the financial cycle.
The sensitivity of the chosen GaR model to data revisions was also evaluated in the context of cross-comparability of stress tests developed in different periods. As is well known, the GDP values are revised from time to time. GDP revisions (vintages) and releases of new information also influence the values of the deflated CCRI.
A comparison of the GDP values was made for the period beginning with the October vintage of 2022, because the deflated CCRI was first calculated in the second quarter of 2022. From that time until September of 2023, GDP has been revised 9 times. For the sake of clarity, Table 1 includes only 4 GDP vintages with the most significant revisions compared to the previous data versions. They approximately correspond to the schedule of the deflated CCRI calculations (see Table 2).
Table 1 shows that the most significant changes in GDP were made between the last vintage at the end of September 2023 and all the other vintages. Notable GDP revisions have also taken place in October 2022 and April 2023. The values of the deflated CCRI change by 0.8–1.8% each quarter. Interestingly, the differences between the deflated CCRI vintages are gradually increasing, while in the case of GDP they are fluctuating.
Recalculation of the GaR model with different data vintages (see Table 3) showed that the influence of data changes is relatively smaller for the time horizon 3 to 6 quarters ahead. In the periods, where data revisions were insignificant (end of 2022, beginning of 2023), the differences between the GDP growth rates do not exceed 0.6 percentage points.
Therefore, when comparing stress test scenarios for different periods, it should be borne in mind that the GaR estimates may change not only because new data have become available, but also because another GDP revision has been done. The value of the GDP growth rate at risk can be as much as 2 percentage points higher or lower due to data revisions alone.
[1] Economists usually mention GDP growth rate forecasts that approximately correspond to the mean value of the GDP growth rate probability distribution.
[2] Percentile is one of the values of a variable that divides the distribution of the variable into 100 groups having equal frequencies (dictionary.com).
[3] For example, Lang, Rusnak & Greiwe (2023), Szendrei & Varga (2023), Chavleishvili & Kremer (2023)
[4] It is planned to extend this period to 3 years.
[5] For more details about the choice of the GaR model, see the technical details' section of this paper.
[6] In econometrics, such variables are called the 'dummy variables' and they take value 1, if a particular condition is met (in this case – periods of Covid-19 crisis), and value 0 in all other cases.
Textual error
«… …»